Many researchers and practitioners talk about AI, IoT, automation, etc, as the Fourth Industrial Revolution (sometimes Industry 4.0) following steam power, electricity, and computerization.
Industry 2.0?
I am more persuaded by the counterarguments. For example, see a post by Luke Muehlhauser arguing there was only one industrial revolution, because the Industrial Revolution was substantively larger than, and different from the others, as exhibited in the figure below.
Yet, there is a sense that what we are seeing now might be similar in scale to the industrial revolution, and contemporary choices we make may play a big role.
Last week (May 2023), Professors Daron Acemoglu and Simon Johnson just published a book, Power and Progress: Our Thousand-Year Struggle Over Technology and Prosperity. There are many econ jokes about Daron’s legendary productivity. (For instance, he wrote the book, probably in the time it took me to write this post).
Here is Daron on the difficult transition it to took to improve society in the decades after Industrial Revolution, and how it cannot be solved automatically.
Second, the Industrial Revolution wasn’t this smooth process via which all of our living standards, or at least the living standards in the West, were lifted up. The first 80 years or perhaps 90 years of the Industrial Revolution brought few benefits for workers in terms of real incomes. So it was a bit like what the peasants experienced during the Medieval period, but actually even worse because their working hours expanded, working conditions worsened, and there was much greater discipline alongside much harsher working conditions. The lives of workers also became poorer because infectious diseases were rampant, and housing and sanitary conditions deteriorated. So the first eight or nine decades of the Industrial Revolution were really bad news for the working classes.
And then something remarkable happened. There was a turnaround, and wages started increasing, working conditions improved, cities were cleaned up, the beginnings of welfare states, health systems and mass education were introduced, and many parts of the West were becoming democratic.
What I view as the essence of techno-optimism is not to deny that there were dark periods of hardship at the beginning of the Industrial Revolution, but to maintain that these were temporary, transitional costs and the improvements were essentially automatic. This optimism clings to the view that we will always find ways of adjusting our institutions and economy, so that most people benefit from technological advances.
This is what I disagree with. Those first eight or nine decades cannot be dismissed as a temporary, transitional period of hardship. There was nothing automatic about it, and we have to understand how political and social struggles changed who benefits from new technologies and the nature and direction of these technologies. Fundamentally, these struggles changed who controlled technology and who decided how the gains from new technology were divided.
Put differently, during the early phases of the Industrial Revolution, working people did not have the power to influence the direction of technology. And they did not have the power to negotiate for better living conditions, better working conditions, or better wages. And that created an environment in which all the gains were captured by a new entrepreneurial class.
Based on his Twitter thread, I break down the five major challenges we face.
AI will create massive inequality.
This will be accompanied by big productivity gains.
Who controls the data? How centralized is the control?
Effect on Democratic politics… which leads to the vexing question of:
How to regulate? Who regulates?
Hard to hit them on all in this weekly essay. So, I will flag the first point and discuss two kinds of jobs at opposite ends of the spectrum of Automation and AI in Operations: (1) Modern Day assembly jobs, and (2) Slow inefficient jobs.
LLMs are Modern-Day Assembly Lines
Much of the AI technology requires a fixed cost of investment in “training” the technology (often addressed under the umbrella of “supervised learning” in Machine Learning). People are excited to hear AI can “learn and play Go by itself”. Still, a large amount of labor-processed data is required for any AI to work well.
For instance, AmTurk uses a significant amount of outsourced labor at cheaper marginal costs, to train AI data such as automatic photo tagging products on a webpage. A 2016 study by TechRepublic reported that the typical weekly wage for AmTurk workers is $79, and 75% of AmTurkers are American. Most tasks are worth a few cents, and as a result, workers complete thousands of repetitive tasks.
The LLMs all come from the need for speed and the supply of billion-dollar investments (some of it under the no-tax benefits of non-profits) to sate its vast appetite for input — by training on trillions of tokens (who owns them?) using more and more compute, consuming energy that can shut down small towns to come up with the next word, like a stochastic parrot. There is despite all this, a vast reasonable excitement. Add to this excitement, human feedback will only make them better. [I love the scholars who are getting famous by the day, by running little tests on LLMs. But this fame is fleeting, we will all be like the unmemorable first guys who improved the Spinning Jenny during the Industrial Revolution].
This scaling improvement is not dissimilar to old ideas of scaling an assembly line and production plant — using division of labor to keep it chugging and making continuous improvements.
Except — we don’t know what these machines do exactly. There are no principles. Only one billion parameters. (On a related note, an entire field of ‘Learning’, I learned is all about parameter adjustments).
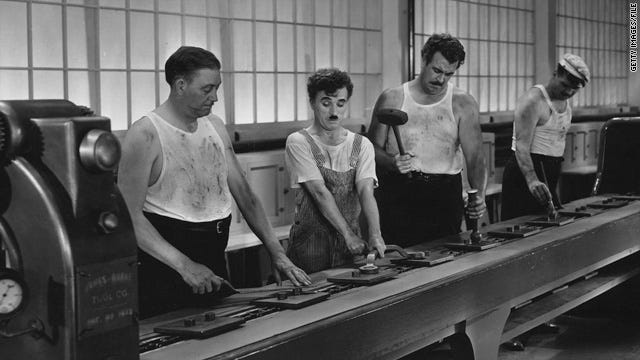
Film aficionados may recall scenes of comic pathos from Charlie Chaplin’s silent classic, Modern Times (1936). The Tramp, played by Chaplin, is unable to keep up with the pace of the assembly line. (How starkly different this approach is, from the concepts of Toyota principle of human-oriented problem-solving at scale?).
In Modern Times, the bosses are testing a machine for making lunches more efficient, by automatically feeding people, leading to one of the most hilarious and poignant scenes in the movie.
Much of the movie follows the Tramp and his Gamine, played by iridescent Paulette Goddard — my favorite scene involves an achingly mesmerizing late-night rendezvous at a department store — the pair roller skating, bumping into things, and adoring closely those trivial items of mass consumption all of which still inevitably far from their hands. In another touching scene, later in the movie, they lay in a park, admiring a distant house they dream of owning, as someone arrives to chase them away.
The Gamine was played by Paulette Goddard — like Chaplin, a loner at heart, and an audacious equal — who chose to go brunette in a Hollywood world populated with platinum blondes, and eventually became his third wife. Even after divorcing Chaplin, she continued to have a stellar movie career of her own, an even more adventurous personal life, and a series of marriages: the Hollywood producer Burgess Meredith, and then the German novelist Erich Maria Remarque, author of the classic book, All Quiet on the Western Front.
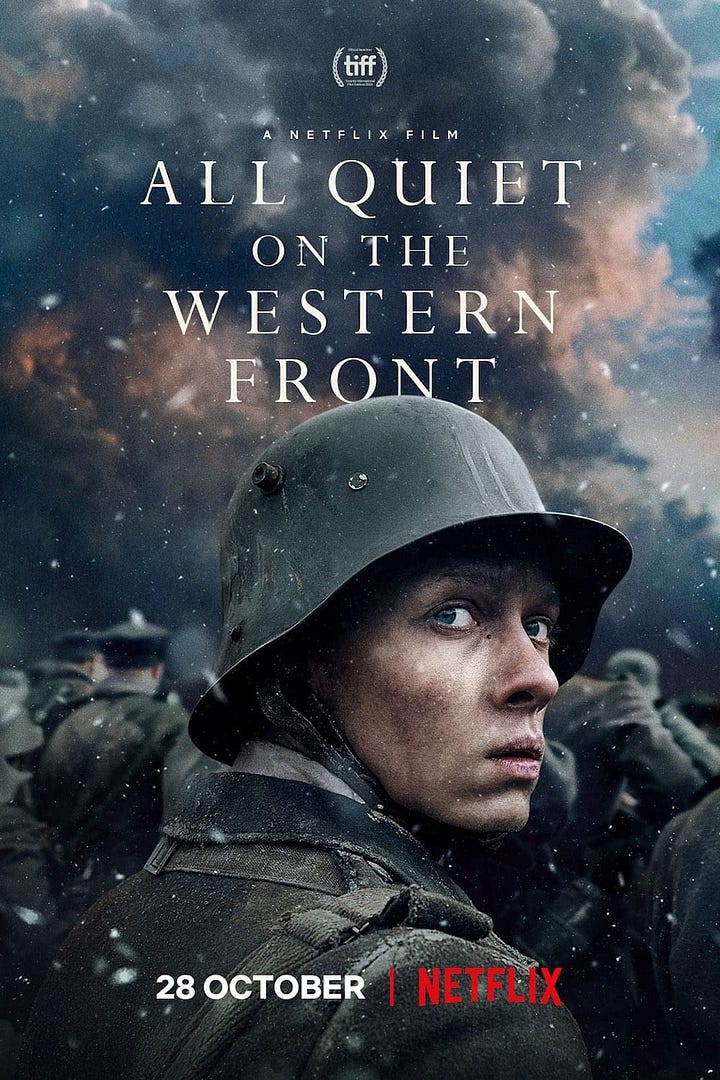
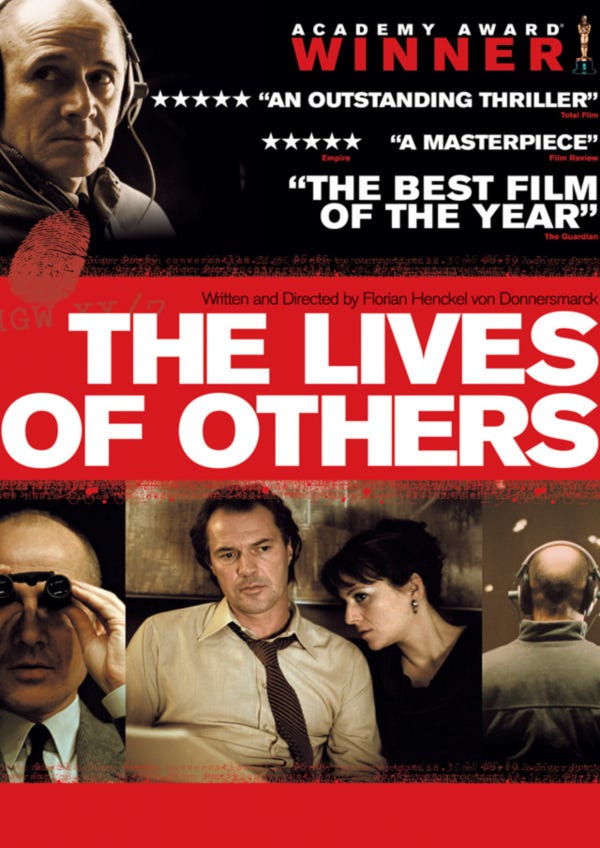
Most researchers concur that AI will create some new kinds of jobs for labor. However, increasingly, we have begun to wonder about how to formulate the production process behind AI training, and the replacement costs of the training labor. The AI, Automation, and Work paper by Acemoglu and Restrepo (2018) builds a model on this issue. I will discuss more frameworks in future posts, but the paper recognizes the vast skill gap that exists between the jobs that train AI/automation and jobs that are created by AI/automation.
The permanent duality of the world is not the fact that for every advance some reap the benefit and some bear the cost — but the society is divided into those who view the costs as natural law, and those who view the costs as an injustice to be corrected.
Jobs Saved by Baumol Cost Disease?
A second class of jobs that had remained relatively untouched by the digitization explosion has now come under assault. In their book, Second Machine Age, researchers Erik Brynjolffson and Andrew McAfee note that some of the jobs that have not been automated are jobs such as gardeners, musicians, front-line workers, and service operations jobs that face consumers.
These jobs possess aesthetic and interactive components and comprise some valued labor content. The economist William Baumol recognized the labor-intensive nature of such jobs and showed that they have low productivity growth.
Think of classical music. We have thousands of discs and music files of recorded concert orchestras, but we still find live concerts are superior. There has not been, and indeed there cannot be, an increase in productivity in concert performances. Here is an analogy originally by James Surowiecki, from the New Yorker (2003)1:
When Mozart composed his String Quintet in G Minor (K. 516), in 1787, you needed five people to perform it—two violinists, two violists, and a cellist. Today, you still need five people, and, unless they play really fast, they take about as long to perform it as musicians did two centuries ago.
Many jobs that are relatively unaffected by AI, are indeed riddled with the cost-disease. Productivity may be lagging in these job sectors, but the cost disease is the saving grace that buys us some time under the AI onslaught.
We can improve productivity by raising the tempo (try that!) or building bigger orchestra halls (imagine being a guy seated in the thousandth row). Both produce poorer outcomes. Sometimes the core class I teach gets oversubscribed, I try to cut the size back to 50. Being the 75th person is not fun — you may never get to participate in discussions — also, I can’t remember names as well. There is famously a quality-speed tradeoff.
Once every few years, when I receive a snarky comment about how a semester’s worth of class can be taught in one or two hours, I think of Baumol. The only gift we can give in the dissemination of information is the labor of love — the time someone willingly spends on a concept.
This is the point I made about the broken life of Toulouse-Lautrec in the birth of the explosion of lithography technology,2 or the childless couple on Mother’s Day. Your life is your product. The fruits of labor and the stories we tell are about the time we put in.
I am sure that with the trillion tokens and hyper-adjusted parameters, LLMs can come up with an essay like this one — maybe even with better phrases and beauty — except it won’t be my words. Writers are now on strike, on TV shows, because AI is quickly replacing those jobs. We forget that the bon mot is interesting not by itself, but the turn of the phrase lies in the interaction with the occasion.
LIFE is a KINTSUGI bowl.
It is always curious to me that LLMs optimize for perfection with the questions — the best response chosen based on the closest among the noisy fit with the parameters of questions — whereas the most interesting thing about our life is how our imperfections stand out.
I have been a fan of Kintsugi bowls — because they try to work with imperfections than eliminate those imperfections.
According to legend, the craft commenced when Japanese shogun Ashikaga Yoshimasa sent a cracked chawan—or tea bowl—back to China to undergo repairs. Upon its return, Yoshimasa was displeased to find that it had been mended with unsightly metal staples. This motivated him to goad the contemporary craftsmen to find an alternative — a more aesthetically pleasing method of repair.
When the tea bowl was eventually returned to the shogun, there were streaks of gold running through it, telling its story, and—the warrior thought—adding to its value and beauty. This method of repair became known as kintsugi.
Kintsugi respects the philosophy of seeing beauty in the imperfect, for without it life is far less interesting.
This world is our broken vase — an imperfect broken world with dreams and lives that we are trying to preserve. So, things cannot be automatically resolved. But, there is one thing I know. We should not be dissuaded by the complexity of the challenge.
Imperfect solutions should be welcomed. Like a Kintsugi bowl — they are a gorgeous and imperfect reflection of our messy lives.
https://www.newyorker.com/magazine/2003/07/07/what-ails-us